PhD Student
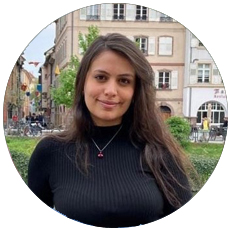
After obtaining my baccalaureate in experimental sciences, I wanted to apply my knowledge to agriculture and environmental issues. Firstly, I obtained my engineering degree in plant health and environment from the National Agronomic Institute of Tunisia (INAT). Later, I decided to specialize in geomatics, so I pursued a research master's degree in geomatics applied to agriculture and the environment at INAT.
Subsequently, I completed my master's internship at the Bureau of Theoretical and Applied Economics (BETA) in Nancy, France, on a subject related to quantifying and mapping ecosystem services. This academic path was followed by a professional experience at BETA as a contractual study engineer for a period of three months.
At CIRED, under the supervision of Benoît Gabrielle and Thierry Brunelle, I will be working on a thesis topic that focuses on locating biomass production for global energy under the constraints of climate and biodiversity. The thesis topic has three main objectives:
i/ estimating land costs, taking into account soil quality (pedology) and competition between land uses (for food, forests, and urbanization), as well as biomass production costs;
ii/ estimating the yield of lignocellulosic biomass crops, taking into account local factors, using a machine-learning approach based on observations made in different regions of the world;
iii/ combining the information obtained from objectives i/ and ii/ to produce regional supply curves and analyze trade-offs between climate regulation and biodiversity, which will be evaluated specifically at this stage.
Localizing Biomass Production for Energy at Global Scale in different climate and energy futures: An Integrated Assessment
A significant increase in the use of biomass for energy is predicted by the vast majority of climate and energy forecasts carried out at national or global level. The increased use of biomass is particularly strong in the most ambitious scenarios in terms of emission reductions (Bauer et al., 2018; IPCC, 2018; IEA, 2021 ; ADEME, 2021). While there is a broad consensus on the role of biomass in future scenarios, the conditions for their implementation remain highly uncertain. Among the various issues raised by the development of biomass energy production, the location of production stands out (Rose et al. forthcoming, Daioglou et al., 2020; Matzenberger et al., 2015). Based on an assessment carried out on a sample of 10 global models, Daioglou concludes that “though most models show increasing bioenergy trade throughout the century in all scenarios, there is significant disagreement on the volumes traded and net importing/exporting regions.” These differences arise from the fact that economic models determine bioenergy supply and demand differently, leading to significant variations concerning the main supply regions and feedstocks (Rose et al., forthcoming). The representation of the bioenergy sector in integrated assessment models relies on the use of supply curves calculated from a combination of agronomic (e.g., bioenergy crop yields) and economic (e.g., harvesting costs, input choice, land price) parameters. A supply curve shows for each quantity offered the corresponding price. Supply curves are generally increasing in price and convex as a result from the assumption of diminishing returns. They are the most suitable tool to determine the choice of technologies considering their production cost. However, the supply curves available in the literature suffer from several important weaknesses:
On the demand side, biomass production and trade will be driven by energy and climate policies. In the most ambitious scenario, bioenergy deployment reaches dramatic scale, with demand on biomass exceeding 300 EJ (Popp et al., 2014). The robustness and value of bioenergy deployment arise from the flexibility to convert biomass feedstocks into various useful energy carriers and the eventual combination with carbon capture and sequestration (CCS), which offsets emissions from fossil fuel use (Bauer et al., 2018). Key drivers of this deployment are (i) the climate policies in place such as the nationally determined contributions (NDCs), (ii) the availability of bioenergy conversion and CCS technologies and other Carbon Dioxide Removal (CDR) options and (iii) the dynamic of the energy system. The possible evolutions of these factors are significant sources of variability on bioenergy deployment. International trade is another key determinant of where biomass for energy is produced. Several recent publications have improved our knowledge of international flows of biomass for energy and the associated transport costs (Daioglou et al., 2020; Hoefnagels et al., 2014; Proskurina et al., 2019). Regarding solid biomass, the main flows are from North America and Eastern Europe to Western Europe. For liquid biomass, the main flows are from South America and South-East Asia to the European Union, Great Britain and some Asian countries (South Korea, Japan). However, this situation will change very quickly with the development of the use of biomass for energy. The objective of this thesis is to better estimate the location of biomass production for energy at global scale within climate/energy scenarios for the 2050/2100 horizon. The work will focus on dedicated energy crop such as woody or grassy bioenergy crops (ligno-cellulosic biomass) and will take into account the main drivers of biomass production location: costs, yields, land availability on the supply side; climate policies, energy, technology availability and macroeconomic context on the demand side. To do so, we will use spatialized datasets and advanced statistical methods to describe the contexts of production. We will also use a global general equilibrium model, IMACLIM-R, to represent the demand side dynamics, including public policies related to climate and energy such as NDCs.
1. Estimates of biomass energy supply curves from spatialized dataThe first objective of the thesis will be to produce supply curves for ligno-cellulosic biomass energy from spatialized data at a resolution of 30 arc-min. We will construct supply curves for 2 types of lingo-cellulosic biomass: woody and grassy energy crops. This work will address the weaknesses of the supply curves available in the literature in 3 main steps. 1.1 Estimating the cost of land taking into the heterogenous quality of landObjective: Estimating the land rental cost according to their main characteristic (suitability of land for agriculture, distance to market, population density...) Method: The cost of land will be estimated on each grid cell from econometric methods by relating the value of land to its geographical and agronomic characteristics. Geographical characteristics relate to the distant to the main markets and harbors. Agronomic characteristics relate to a set of climatic, soil and topographic indicators. We will rely on a sample of about 150 observations of land costs in major regions of the world (Domingues et al., In Review) and on data from Zabel et al. (2014) on land suitability for agriculture and Verburg et al. (2011) regarding land accessibility. In case econometric methods do not produce significant results, several back-up strategies will be considered. For example we can use estimates of land value that are available in the SPAM database (Yu et al., 2020). 1.2 Estimating the yield of lignocellulosic biomass crops taking into account local factorsObjective: To estimate the yields of several species dedicated to bioenergy as a function of soil and climate characteristics, and crop management practices. One of the main challenges is to account for the effect of soil properties (in particular under marginal conditions) on these yields. Method: We will train a yield prediction model using machine-learning techniques (such as Random Forest and quantile regression Random Forest, following the method proposed by Su et al., 2021 for conservation agriculture). Data will come from databases of published articles (Lu et al., 2018) and supplemented with new articles and missing data (in particular the database resulting from the H2020 project MAGIC involving marginal conditions, 12 crops and 30 locations, Von Cossel et al., 2019). An emphasis will be placed on data obtained in commercial fields versus experimental plots which lead to higher yield estimates and may be biased (Searle and Malins, 2014) 1.3. Producing dedicated energy crop maps and associated supply curves based on a large sample of biomass production costObjective: Producing dedicated energy crop maps and associated supply curves using the land rental cost and biomass yields previously calculated. Method: The cost of biomass for energy will be calculated in each grid cell by dividing the sum of the land rental cost and other production costs (capital, labor, fertilizers) expressed in $ per hectare by the biomass yields in gigajoule per hectare. The other costs will be estimated from the production cost database for biomass energy produced in a previous CLAND project (Domingues et al., In Review). This database compiles 410 observations in 46 countries around the world. The costs will be distributed uniformly over all grid cell in a given country. The costs will be applied to recent global land use maps. Different constraints in the deployment of biomass over land areas will be considered according to criteria of carbon stock in soils and vegetation and/or biodiversity preservation. The production costs calculated at the grid cell will be then ordered in ascending order to build the supply curves in each region. We will consider possibilities for costs to evolve over time as a function of capital/labor substitution (mechanization) and as a result of a learning rate that reflects the increase in productivity with experience. The spatialized supply curves that we will produce here will allow us to estimate precisely the location of biomass production and to assess the associated effect the carbon stocks in soil and vegetation. 2. Integration of supply curves in Imaclim-RThe Imaclim-R model (Sassi et al., 2010; Waisman et al., 2012) is a multi-region and multi-sector model of the world economy. It combines a Computable General Equilibrium (CGE) framework with bottom-up sectoral modules in a hybrid and recursive dynamic architecture. Furthermore, it describes growth patterns in second best worlds with market imperfections, partial uses of production factors and imperfect expectations. Bioenergy technologies were formerly integrated into Imaclim-R by (Bibas and Méjean, 2013) through two distinct external supply curves taken from Hoogwijk al. (2009) for bio-electricity and from IEA (2006) for biofuels. These supply curves were expressed in secondary energy terms. Objective: The objective here will be to replace the Hoogwijk supply curves by those we will have calculated in the previous step. The integration in Imaclim-R will be based on a representation of the bioenergy sector in two stages: primary energy and secondary energy, i.e. the energy obtained after transformation processes in the form of fuels, electricity, hydrogen, gas or heat. Method: The cost and efficiency of converting primary energy into secondary energy will be taken from coefficients from the literature and coefficients already implemented in IMACLIM-R. The main energy carriers considered will be: 2nd generation biofuels, bioelectricity, heat, biogas and biohydrogen. Each technology can be associated or not with CCS using coefficients already implemented in IMACLIM-R. The main challenge will be to represent the trade of biomass in primary energy (the trade in secondary energy is already integrated in Imaclim-R). Different simple trade models will be considered: relative price, gravity models etc. If time permits, we may consider using more complex models, such as the Biomass Intermodal Transportation Model (BIT-UU, Hoefnagel et al., 2014). This model has the advantage of calculating intermodal transport routes (road, rail, Inland waterways, sea, ocean) that are optimized for lowest cost. This model will also provide us with the detailed transport data and uses of biomass, which will be useful to estimate accurate GHG emissions from transport, as incorporated in bioenergy value-chains. 3. Energy and Climate policy simulation in Imaclim-RThree important dimensions are affecting the demand for bioenergy at global scale. First, the future availability of advanced technologies for bioenergy and CCS affects both the cost and carbon efficiency of bioenergy (Bauer et al., 2018). The development of CDR options will also impact BECCS demand. Indeed, CDR is essential to achieve net zero emission targets, and in the worst-case scenario to compensate for emissions overshoot. In IAM, BECCS tend to be the only option implemented for CDR, sometimes with afforestation. However, other negative emission option are currently under research and development, such as direct air carbon capture and storage (DACCS) and nature-based CDR options (enhanced weathering, biochar etc.) (Minx et al., 2018). Uncertainties remain large as to their scaling potentials and environmental impacts, but their future deployment is likely to reduce the dependency on BECCS (Köberle, 2019). Second, the evolution of the global energy system can also significantly affect bioenergy demand. As biofuels are a substitute to fossil fuels, the dynamic of fossil reserve extraction may be a key driver of biofuels development. For instance, a rapid oil rarefaction due to a decreasing profitability of exploitation or stringent policies on the preservation of fossil carbon stocks may foster demand for liquid biofuels. Future innovations on other renewable energies and their grid penetration would also have complex consequences on bioenergy demand (Köberle, 2019). Third, the trajectory of climate mitigation, and especially the level of residual emissions to compensate with negative emissions, set the scale and temporality of bioenergy deployment. Increasing bioenergy deployment becomes generally more important for more stringent emission budgets. Particularly, achieving tighter budgets boost bioenergy deployment by 2050 (Bauer, 2019). Objective: To investigate the influence of policies, technologies and energy dynamics on bioenergy and biomass demand. To this aim, we will simulate different contrasted climate change mitigation and energy scenarios, and analyse the implications in terms of i) bioenergy deployment (magnitude, energy vectors, CCS), ii) international trade of bioenergy and localization of supply, and ii) macro-economics impacts. From this analysis we will be able to assess the range of plausible bioenergy deployment, to identify the main drivers of bioenergy and biomass demand, and to evaluate the related uncertainties. Method: The method will rely on the Imaclim-R model to produce climate mitigation and energy scenarios. Imaclim-R is especially suited to simulate these energy system evolutions, as it integrates relevant market imperfections (underutilisation of capacities, investment decisions based on limited forecast capability, and inertia in technological changes). The uncertainty on the different parameters influencing bioenergy demand will be a key point of the analysis. We will explore here large ranges of scenarios that we will analyze on the basis of dedicated scenario techniques such as robust decision making or scenario discovery (Guivarch et al., 2017). The scenarios will be built to cover a large range of plausible futures, addressing three dimensions refering to policies, bionergy and CDR technologies, and energy dynamics. To simulate different policies, the scenario will consider nationally determined contributions (NDCs) and more ambitious climate mitigation scenarios. Most of these scenarios are already implemented in Imaclim-R, and will be updated according to sixth IPCC assessment report, updated NDCs published by countries and other major research publications. Different schemes of carbon taxation associated with different regimes of international cooperation will also be examined. In particular we will assess fragmented international climate policies. The plausible and extreme trends for key technical drivers will then be integrated in the scenario (cost and availability of advanced bioenergy, CCS technologies etc.). A few adjustment in Imaclim-R modules will be necessary, especially for the modelling of CDR options. A simple DACCS model will be added, and other CDR options will also be integrated at a very aggregated level, based on the literature on cost, potentials and future technical improvement (Fuss et al., 2018; Nemet et al., 2018; Chiquier et al., forthcoming). In the context of this study, this relatively simplified modelling should be sufficient to simulate CDR options. Dynamics in fossil energies exploitation and renewable energies development will eventually be integrated in scenario design. We will explore here different fossil resource depletion futures through a range of assumptions on the shape of Hubbert curves (the Hubbert curve is an approximation of the production rate of a given resource over time. It is a symmetric logistic distribution curve). The analysis of the results of the scenarios will focus on (i) the deployment of bioenergy (evolution and magnitude, repartition between the different energy vectors, proportion of CCS, etc.), (ii) the induced repercussions on biomass production and trade (scale, localisation, costs, transports) and (iii) the macro-economic impacts (trade balance, energy costs, carbon price, GDP, employment, etc.). If time permits, we also consider different scenarios regarding the sustainability of biomass production. We will consider here criteria related to land carbon stocks, biodiversity impacts and land access rights. ConclusionThe main expected results of this thesis will be (i) to produce spatialized estimates of cost effective biomass production in a wide range of climate and energy scenarios ; (ii) to estimate associated GHG emissions in relation to carbon dynamics and storage in soils and vegetation, as well as international transportation of biomass ; (iii) from a policy perspective, to better assess the role of bioenergy for climate change mitigation, taking into account local constraints on the development of biomass production and indirect effects on land-use and GHG emissions. This thesis will also broaden our understanding of the interactions between bioenergy and mitigation policies. It will reveal the economic advantages of bioenergy or BECCS deployment as well as the limits and risks compared to other mitigation options. Results may be relevant for robust and resilient policy design on bioenergy development and biomass production. Indeed, the prospect of relying on BECCS to generate negative emissions in the future may delay efforts on emissions reductions, and this work will help quantify the risks if BECCS is less carbon and cost efficient as expected. The evaluation of the potential and cost of late BECCS deployment will also cast light upon the risks of failing to achieve mitigation policies. This thesis will finally make a significant contribution to the literature by providing biomass supply curves with improved costs and yields estimates, taking into local properties of soil affecting biomass yields as well as the great heterogeneity in land quality affecting land costs. ReferencesBauer, N., Rose, S.K., Fujimori, S., van Vuuren, D.P., Weyant, J., Wise, M., Cui, Y., Daioglou, V., Gidden, M.J., Kato, E., Kitous, A., Leblanc, F., Sands, R., Sano, F., Strefler, J., Tsutsui, J., Bibas, R., Fricko, O., Hasegawa, T., Klein, D., Kurosawa, A., Mima, S., Muratori, M., 2018. Global energy sector emission reductions and bioenergy use: overview of the bioenergy demand phase of the EMF-33 model comparison. Climatic Change. https://doi.org/10.1007/s10584-018-2226-y Bibas, R., Méjean, A., 2013. Potential and limitations of bioenergy for low carbon transitions. Climatic Change 1–31. https://doi.org/10.1007/s10584-013-0962-6 Daioglou, V., Rose, S.K., Bauer, N., Kitous, A., Muratori, M., Sano, F., Fujimori, S., Gidden, M.J., Kato, E., Keramidas, K., Klein, D., Leblanc, F., Tsutsui, J., Wise, M., van Vuuren, D.P., 2020. Bioenergy technologies in long-run climate change mitigation: results from the EMF-33 study. Climatic Change. https://doi.org/10.1007/s10584-020-02799-y Fuss, S., Lamb, W.F., Callaghan, M.W., Hilaire, J., Creutzig, F., Amann, T., Beringer, T., Garcia, W. de O., Hartmann, J., Khanna, T., Luderer, G., Nemet, G.F., Rogelj, J., Smith, P., Vicente, J.L.V., Wilcox, J., Dominguez, M. del M.Z., Minx, J.C., 2018. Negative emissions—Part 2: Costs, potentials and side effects. Environ. Res. Lett. 13, 063002. https://doi.org/10.1088/1748-9326/aabf9f Guivarch, C., Lempert, R., Trutnevyte, E., 2017. Scenario techniques for energy and environmental research: An overview of recent developments to broaden the capacity to deal with complexity and uncertainty. Environmental Modelling & Software 97, 201–210. https://doi.org/10.1016/j.envsoft.2017.07.017 Hoefnagels, R., Searcy, E., Cafferty, K., Cornelissen, T., Junginger, M., Jacobson, J., Faaij, A., 2014. Lignocellulosic feedstock supply systems with intermodal and overseas transportation. Biofuels, Bioproducts and Biorefining 8, 794–818. https://doi.org/10.1002/bbb.1497 Hoogwijk, M., Faaij, A., de Vries, B., Turkenburg, W., 2009. Exploration of regional and global cost–supply curves of biomass energy from short-rotation crops at abandoned cropland and rest land under four IPCC SRES land-use scenarios. Biomass and Bioenergy 33, 26–43. https://doi.org/10.1016/j.biombioe.2008.04.005 Intergovernmental Panel on Climate Change, 2018. Global warming of 1.5°C. Köberle, A.C., 2019. The Value of BECCS in IAMs: a Review. Current Sustainable/Renewable Energy Reports 6, 107–115. https://doi.org/10.1007/s40518-019-00142-3 Matzenberger, J., Kranzl, L., Tromborg, E., Junginger, M., Daioglou, V., Sheng Goh, C., Keramidas, K., 2015. Future perspectives of international bioenergy trade. Renewable and Sustainable Energy Reviews 43, 926–941. https://doi.org/10.1016/j.rser.2014.10.106 Minx, J.C., Lamb, W.F., Callaghan, M.W., Fuss, S., Hilaire, J., Creutzig, F., Amann, T., Beringer, T., Garcia, W. de O., Hartmann, J., Khanna, T., Lenzi, D., Luderer, G., Nemet, G.F., Rogelj, J., Smith, P., Vicente, J.L.V., Wilcox, J., Dominguez, M. del M.Z., 2018. Negative emissions—Part 1: Research landscape and synthesis. Environ. Res. Lett. 13, 063001. https://doi.org/10.1088/1748-9326/aabf9b Nemet, G.F., Callaghan, M.W., Creutzig, F., Fuss, S., Hartmann, J., Hilaire, J., Lamb, W.F., Minx, J.C., Rogers, S., Smith, P., 2018. Negative emissions—Part 3: Innovation and upscaling. Environ. Res. Lett. 13, 063003. https://doi.org/10.1088/1748-9326/aabff4 Popp, A., Rose, S.K., Calvin, K., Van Vuuren, D.P., Dietrich, J.P., Wise, M., Stehfest, E., Humpenöder, F., Kyle, P., Van Vliet, J., Bauer, N., Lotze-Campen, H., Klein, D., Kriegler, E., 2014. Land-use transition for bioenergy and climate stabilization: model comparison of drivers, impacts and interactions with other land use based mitigation options. Climatic Change 123, 495–509. https://doi.org/10.1007/s10584-013-0926-x Proskurina, S., Junginger, M., Heinimö, J., Tekinel, B., Vakkilainen, E., 2019. Global biomass trade for energy— Part 2: Production and trade streams of wood pellets, liquid biofuels, charcoal, industrial roundwood and emerging energy biomass. Biofuels, Bioproducts and Biorefining 13, 371–387. https://doi.org/10.1002/bbb.1858 Sassi, O., Crassous, R., Hourcade, J.-C., Gitz, V., Waisman, H., Guivarch, C., 2010. IMACLIM-R: a modelling framework to simulate sustainable development pathways. International Journal of Global Environmental Issues 10, 5–24. https://doi.org/10.1504/IJGENVI.2010.030566 Searle, S.Y., Malins, C.J., 2014. Will energy crop yields meet expectations? Biomass and Bioenergy 65, 3–12. https://doi.org/10.1016/j.biombioe.2014.01.001 Su, Y., Gabrielle, B., Makowski, D., 2021. The impact of climate change on the productivity of conservation agriculture. Nat. Clim. Chang. 11, 628–633. https://doi.org/10.1038/s41558-021-01075-w Verburg, P.H., Ellis, E.C., Letourneau, A., 2011. A global assessment of market accessibility and market influence for global environmental change studies. Environmental Research Letters 6, 034019. Von Cossel, M., Lewandowski, I., Elbersen, B., Staritsky, I., Van Eupen, M., Iqbal, Y., Mantel, S., Scordia, D., Testa, G., Cosentino, S.L., Maliarenko, O., Eleftheriadis, I., Zanetti, F., Monti, A., Lazdina, D., Neimane, S., Lamy, I., Ciadamidaro, L., Sanz, M., Esteban Carrasco, J., Ciria, P., McCallum, I., Trindade, L.M., Van Loo, E.N., Elbersen, W., Fernando, A.L., Papazoglou, E.G., Alexopoulou, E., 2019. Marginal Agricultural Land Low-Input Systems for Biomass Production. Energies 12. https://doi.org/10.3390/en12163123 Waisman, H., Rozenberg, J., Sassi, O., Hourcade, J.-C., 2012. Peak Oil profiles through the lens of a general equilibrium assessment. Energy Policy 48, 744–753. Yu, Q., You, L., Wood-Sichra, U., Ru, Y., Joglekar, A.K.B., Fritz, S., Xiong, W., Lu, M., Wu, W., Yang, P., 2020. A cultivated planet in 2010 – Part 2: The global gridded agricultural-production maps. Earth Syst. Sci. Data 12, 3545–3572. https://doi.org/10.5194/essd-12-3545-2020 Zabel, F., Putzenlechner, B., Mauser, W., 2014. Global Agricultural Land Resources – A High Resolution Suitability Evaluation and Its Perspectives until 2100 under Climate Change Conditions. PLOS ONE 9, e107522. https://doi.org/10.1371/journal.pone.0107522 |
Name of the applicant (PhD director)
Benoit Gabrielle (AgroParisTech/ECOSYS), Thierry Brunelle (CIRAD/CIRED), Julien Lefevre (AgroParisTech/CIRED) |
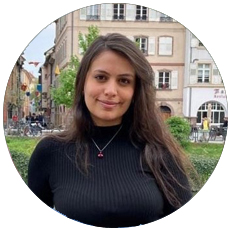
Siwar Saadaoui joins the CarMa Research Team
November 22, 2022